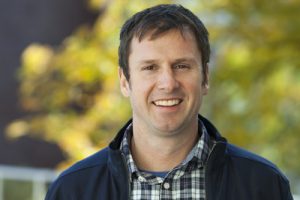
Until very recently, learning about new music carried a human element. Whether you heard something on college radio that peaked your interest, or someone cornered you at a wedding, insisting that you had to hear a great new band, you received recommendations originating with people. Recently, computer algorithms have taken on this task, sifting and refining consumer demographics and listening habits into recommendations. When you stream three Marvin Gaye albums in a row on Spotify, it’s probably not surprising that the service next suggests Bruno Mars. But as algorithms increasingly influence how we consume music, researchers like Professor Jeremy Morris are interested in the whys and hows of this interaction. He is also concerned about their impact on artists, the music industry, and consumers.
In 2019, Professor Morris won a grant to co-lead AI, Recommendations & the Curation of Culture, a workshop assembling business and media scholars, computer scientists, artists, and music industry representatives to brainstorm on the topic. The 3-day workshop generated many interesting discussions that Morris and his colleagues Georgina Born (Oxford University), Ashton Anderson (University of Texas), and Fernando Diaz (Google) expanded to an in-depth report “Artificial Intelligence, Music Recommendation, and the Curation of Culture,” published by the Schwartz Reisman Institute.
The report considers almost every step of the music industry’s processes, from the ways in which algorithms manipulate the existing variety of content itself, to how it is produced, distributed, valued, and consumed around the world. A few of the important questions explored include:
- What assumptions are built into media recommendation systems?
- How does an algorithm’s “understanding” of music and of music listeners shape the recommendations we are presented?
- Does extreme personalization work at odds with the diversity and variety of local and regional music cultures that may not be accurately reflected through global streaming services?
Professor Morris is also interested in strategies artists may adopt to “jump the queue” so to speak, whether to garner more fans, or generate more plays of their songs, which directly impacts their earnings. “Algorithmic recommendation systems not only influence what consumers are presented, they have direct effects on the type of songs that artists produce to generate fans, listens, and plays on platforms like Spotify. They can also result in artists adjusting their songs and metadata of their tracks, in order to appear higher up in search results and have their music be more ‘platform ready’,” he notes.
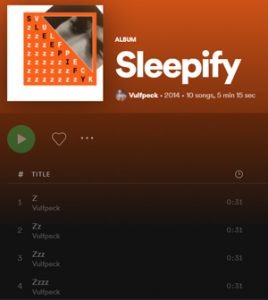
As an example of this type of manipulation, he mentions the clever case of funk band Vulfpeck, who created an album of silent tracks which hovered around the minimum duration (30 seconds) that would count as played and net a royalty. Exploiting a loophole in how the Spotify streaming service calculated earnings, the band encouraged consumers to play the album, “Sleepify,” on a loop while they slept, ultimately garnering about $20,000 before company leadership intervened.
Morris and his colleagues are also concerned that recommendation systems can perpetuate the dominance of music that is already popular. He reflects that it’s hard for independents to compete with well-funded artists like Taylor Swift, and that the ubiquity and popularity of platforms like Spotify and Pandora can discourage competing services.
Of course, artists have long had to contend with (human) gatekeepers, trying to get their music recognized by DJs, A&R (Artist & Repertoire), or venue owners. Even as their algorithms may favor established artists, platforms such as Spotify and Pandora provide an opportunity to bypass gatekeepers and present music directly to potential fans. Just as with earlier platforms, whether FM radio or Napster, Morris sees opportunity and challenge for artists and consumers alike.